Published:
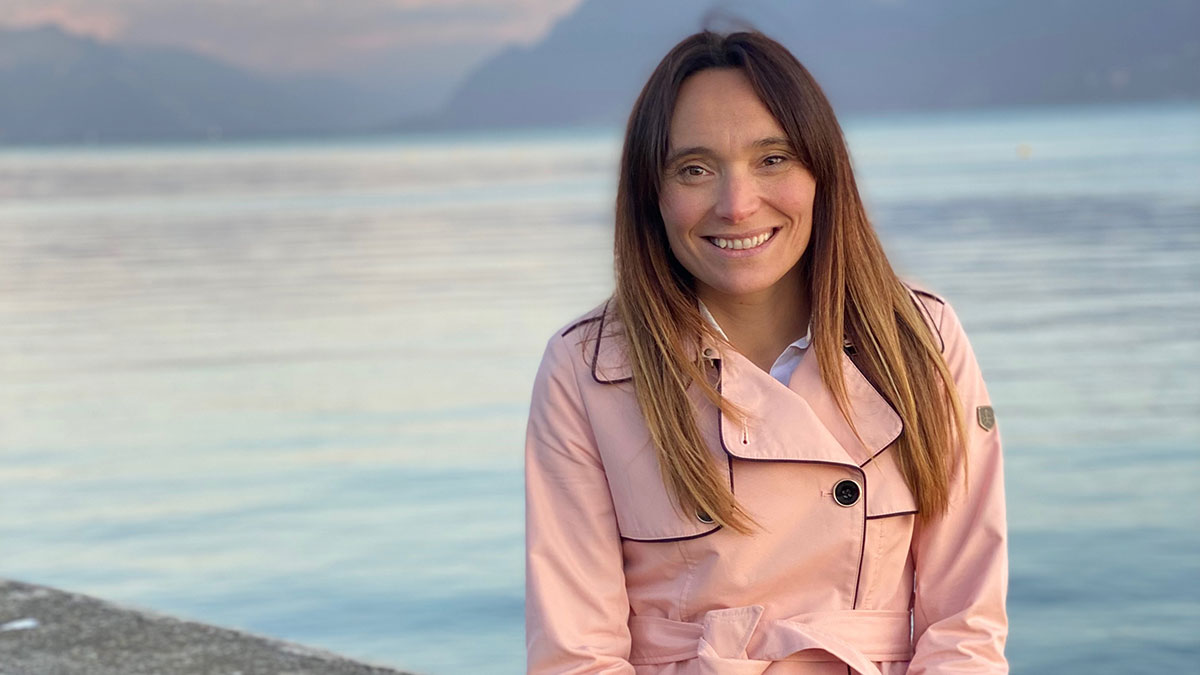
Chemical engineers at Heriot-Watt University have contributed to developing a machine-learning model that accurately predicts the heat capacity of metal-organic frameworks and other adsorbents.
The study, published today in Nature Materials, opens exciting opportunities for future carbon capture technologies.
Metal-organic frameworks (MOFs) are a class of materials that contain nano-sized pores.
These pores, as well as the rich and varied chemistry of MOFs, make them extremely versatile and promising for several applications, including capturing carbon dioxide (CO2) from power and industrial plants.
Susana Garcia, a Professor of Chemical and Process Engineering and the Associate Director in Carbon Capture and Storage at the Research Centre for Carbon Solutions (RCCS), is advancing the possibilities around novel materials through her research that includes MOFs and separation processes for energy, industrial and environmental applications.
She is currently leading the ACT-funded PrISMa project, where a consortium of international researchers has developed a technology platform to accelerate the deployment of carbon capture technologies through the integration of process engineering and basic science.
“The results of the PrISMa platform have showcased the importance that materials properties like the heat capacity have for estimating the energy required to drive a carbon capture process and have triggered the need for this study,” said Prof. Garcia.
“This is about predicting how much energy is needed to heat a material by one degree.”
Prof. Garcia's team also uses the platform to decarbonise the UK industry as part of the efforts within the UK Industrial Decarbonisation Research and Innovation Centre – IDRIC.
To demonstrate the real-world impact of their work, the team simulated the performance of the materials in a carbon capture plant. Dr. Charithea Charalambous, research associate in Prof. Garcia's team and technical lead of PrISMa's modelling work, developed the process model.
Dr. Charalambous, explains: “Our results show that with correct heat capacity values of MOFs, the overall energy cost of the carbon capture process is much lower than we originally calculated.”
“For some MOFs, this was a 50% reduction of the energy costs, which greatly impacted the process's techno-economic viability.”
Until now, all engineering calculations assume that all MOFs have the same heat capacity for the simple reason that there is hardly any data available. The surprising aspect of this work is that Professor Berend Smit's team at École polytechnique fédérale de Lausanne, a public research university in Switzerland, used a big-data approach for a problem where there was no data.
They realised that one could machine learn how the local chemical environment impacts the vibrations of each of the atoms present in a MOF and these vibrations can be related to the heat capacity. To test the predictions, the researchers synthesized several MOFs and measured their heat capacity, which all agreed well with the model's predictions.
Prof. Garcia, finished: “This work highlights the importance of multi-scale and multidisciplinary research efforts in the fight against climate change, and it showcases how artificial intelligence can accelerate solving urgent problems.”